How Artificial Intelligence Improves Worker Performance
With the huge emphasis on Industry 4.0 and the Internet of Things (IoT), manufacturers are keenly aware of using automation, machine learning and big data to improve the quality of their operations. However, there has been limited application of Industry 4.0 technologies for evaluating and improving the performance of human workers, who conduct about 75 percent of the tasks on the factory floor. Tools that are currently used to measure human performance are woefully inadequate, often inaccurate and commonly performed by hand.
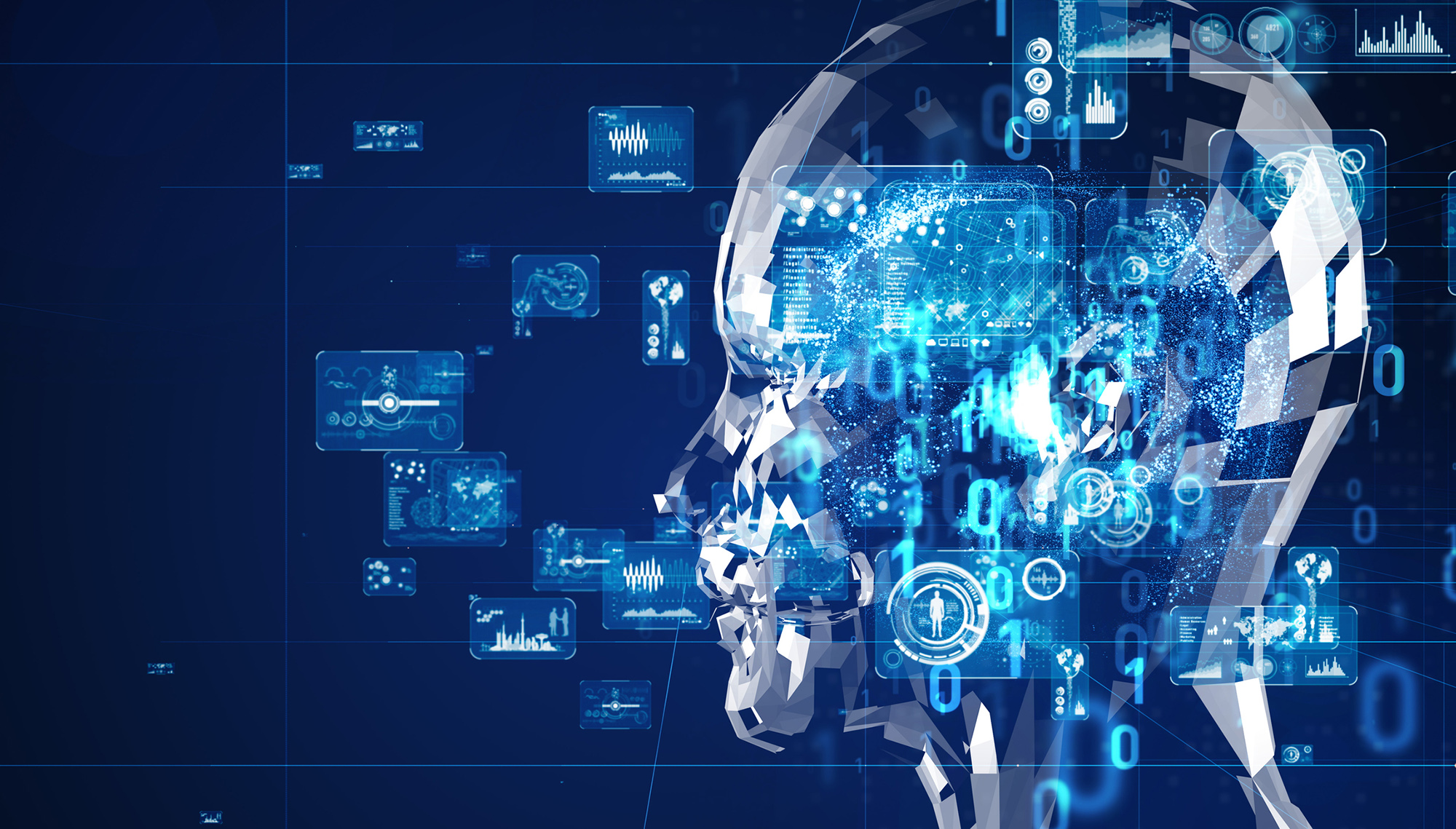
A recent survey by A.T. Kearney and Drishti of 100 manufacturing leaders focused on the manufacturer efforts to measure tasks performed by its employees, as well as the challenges they faced in collecting reliable metrics. The survey revealed that:
- About 72 percent of all factory tasks are performed by humans.
- Humans create 71 percent of value in an operation, compared to robots.
Human performance represents a large amount of operational influence that, for most manufacturers, has not been effectively analyzed. Very few digital methods exist for capturing large volumes of data that represent these human actions, and the lack of accurate analytics makes it difficult for managers to make fully informed decisions.
“Without sufficient analytics on tasks performed by humans, it’s exponentially harder to mitigate variability and trace defects to the source,” states the Kearney report. Because 73 percent of the variability on the factory floor comes from human workers, not machines, developing better analytics is crucial for maximizing operational efficiency, productivity and quality.
Unfortunately, most manufacturers still rely on outdated and inexact measurement techniques. According to the report, 71 percent of respondents identified time and motion studies as important (although often flawed) data; however, conducting these studies consumes nearly 40 percent of an engineer’s time. Root-cause analysis (RCA) is another time-intensive process that takes nearly the same amount of staff time, consuming more than 60 hours a month.
“A significant portion of our industrial engineers’ time is spent on non-value-added work— specifically, gathering and analyzing data through manual means,” indicates Raja Shembekar, director of process and research development at Denso, a global automotive components manufacturer. “This is overhead and does not add value.”
An Industry 4.0 Approach
Artificial intelligence (AI) and machine learning can generate high-value data regarding human performance, which is especially important for identifying and correcting the causes of variability in human tasks. This data is generated in much more depth, and in far less time and without human bias, compared to manual methods, providing HR and floor managers with fast access to valuable and systematized insights. AI makes it possible to track employee engagement and analyze the success of yearly or monthly goals of employees. For example, a UK-based company called WorkCompass states AI can increase employee productivity by 10 percent or more by monitoring performance throughout the year, rather than relying on two six-month performance reviews.
AI can also speed up RCA studies, which are still often done manually by process, quality and maintenance engineers, consuming valuable time. Another disadvantage of manual RCA is the conclusions are typically “not shared across manufacturing sites, leaving factories/plants of the same company to repeat each other’s mistakes, leading to unplanned downtime that could have been prevented,” says Lior Kitain, marketing manager for Seebo, a provider of industrial IoT platforms.
Automated AI and machine methods for conducting RCA are unbiased and based upon historic and real-time data from the production floor. “The ability of AI to formulate predictions relating to machine performance and health, instead of waiting for disaster to strike, introduces a whole range of benefits that affect the bottom line,” continues Kitain. “A data-driven automated RCA system that is accurate and predictive, and offers actionable insights, can be shared between cooperating facilities.”
In the construction industry, AI is being used to improve ergonomics of workers. A recent study by the University of Waterloo shows that motion sensors and AI can determine the safest and most productive ergonomics for bricklaying. For example, master masons employ movements such as swinging blocks, rather than lifting them, which enable them to work twice as fast with half the effort, and a much lower risk for injury. “Our team continues to look at ways that AI can extract safe postures that expert workers use to perform work safely and effectively, as a first step towards creating a training tool for novice workers,” says study leader Abdullatif Alwasel, a professional engineer with a specialty in human kinematics.
Moving Forward
Ultimately, AI can help HR personnel and floor managers streamline tasks and gain unprecedented insights into the real performance potential of employees, notes Jeremy Nunn, founder and director of Workmetrics, a provider of workforce software. “This is all done without the limitations of human bias and capacity for error, making AI a HR management tool of the future, with benefits already ripe for the picking,” he says.
Some opinions expressed in this article may be those of a contributing author and not necessarily Gray.