Four Use Cases for AI's Growing Role in Manufacturing
Artificial intelligence has passed its first test. Driven by sophisticated algorithms and an insatiable appetite for data, the technology has proven its capacity to disrupt industries with its talent for synthesizing information into actionable insights. As industry leaders shift their questions about AI from “Should we?” to “How can we?”, the biggest uncertainty lies in finding a use case that’s impactful, efficient, and practical.
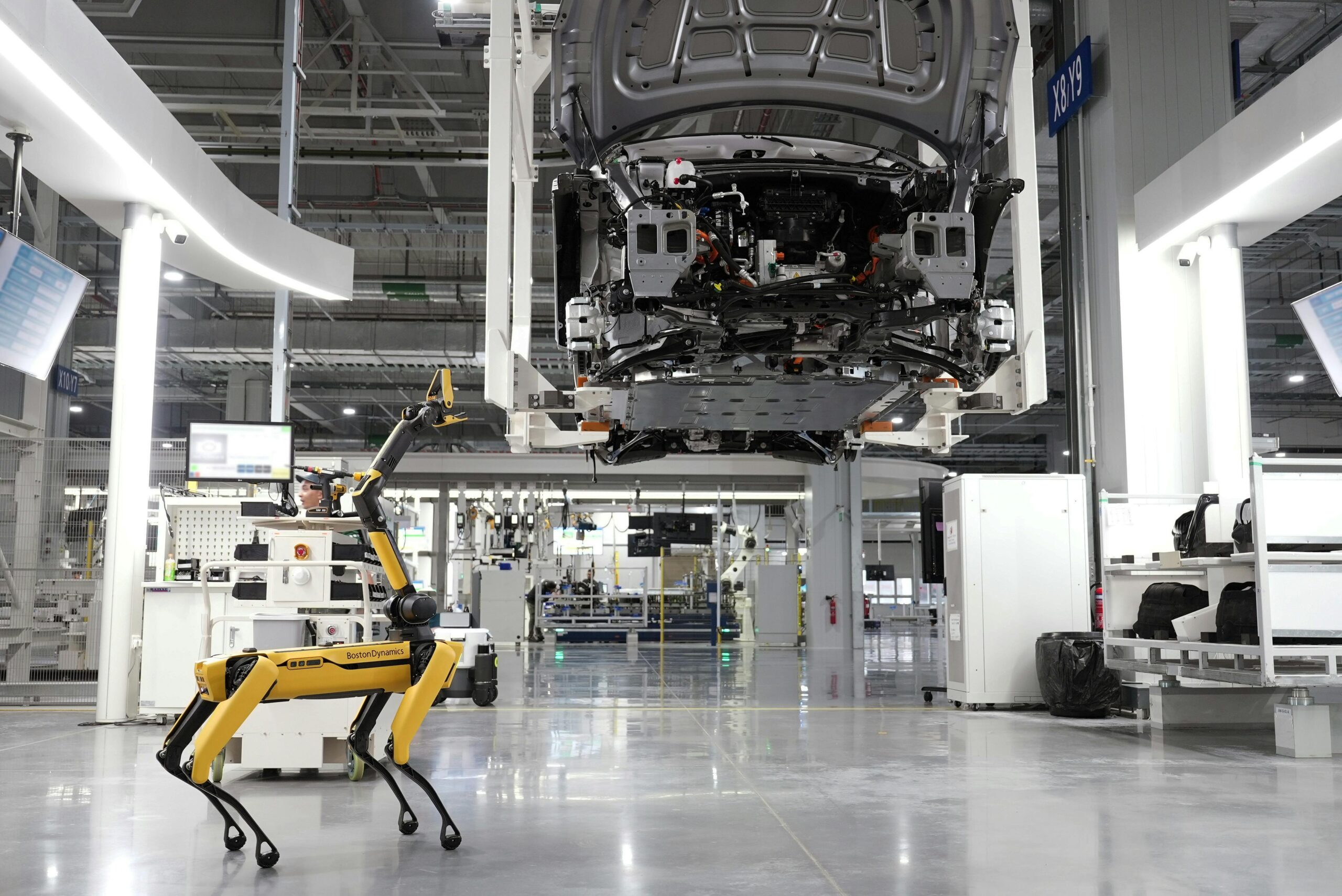
How manufacturers deploy AI varies greatly—those leery of giving it too much influence over operations may relegate it to the back office as a soft-skill, administrative tool. The more intrepid may plan for advanced uses, such as self-correcting “lights out” manufacturing that runs 24/7 without the need for onsite supervision.
However the use, manufacturers’ goals are always the same: higher productivity, greater quality, and lower costs, which in turn lead to happier customers and bigger market share.
Let’s look at four emerging use cases of AI in manufacturing. In each instance, the use of AI has been evaluated according to 1) its potential impact on operations; 2) the ease of implementation; 3) the required skilled labor support; and 4) the technology’s current viability/availability. These insights are supported by Dean Elkins, senior director for robotics and vision, and Samuel Janes, manager of technology advancement team, of Gray Solutions, a systems integrator that provides innovative business solutions by using the latest automation technologies.
Use Case 1: Cobots and AMRs
Potential impact on operations
To mitigate labor shortages and achieve efficiency gains, manufacturers are starting to pair cobots and automated mobile robots (AMRs) within the same automation platform for applications that include the transfer of goods within a manufacturing facility, or from one manufacturing operation to another. Once programmed, these devices facilitate the transfer of items, making an operation far less dependent upon human labor and traditional conveyor systems. AI can more quickly tie applications together, referencing historical data and equipment specs to refine programming and find more efficient work paths.
Ease of implementation
AMRs are often taught using Light Detection and Ranging (LIDAR) devices, coupled with fleet management software. AMR missions can usually be tied into a company’s manufacturing scheduling software. AMRs are also equipped with machine vision to facilitate accurate navigation and location in a docking area. Cobots can be taught either through a teach pendant or hand guidance for easy training. If coupled with its own vision system, a cobot can locate and manipulate randomly placed parts effectively.
Required skilled labor support
While ease-of-use tools have simplified the implementation of cobots and AMRs, best practice still dictates that skilled workers attend trainings offered by the OEM or supplier to ensure a smooth startup. These devices are not “plug and play” right out of the box; they require clear but flexible programming and a thorough understanding of the operational environment.
Current viability/availability
In recent years, cobots and AMRs have become much more mature in the development cycle, and a growing number of robotics companies offer multiple platforms with a variety of payload options. Furthering this trend, OEMs are increasingly designing for compatibility to manufacturing software, making these platforms more relevant to a wider range of industrial environments.
Use Case 2: Forecasting to Optimize the Supply Chain
Potential impact on operations
AI-driven forecasting can drastically improve supply chain efficiency by reducing stockouts, minimizing excess inventory, and optimizing production schedules. It empowers manufacturers to make better informed, more proactive decisions by providing actionable insights into demand trends, supplier performance, and market fluctuations. This can result in cost savings, improved customer satisfaction, and greater agility in responding to disruptions.
Ease of implementation
The implementation of AI into demand forecasting is moderately challenging. While off-the-shelf AI tools are available, integrating them with existing enterprise resource planning (ERP) systems and supply chain management platforms can be complex. Additionally, the quality of implementation depends heavily on the availability of clean, historical data and the organization’s willingness to adapt processes based on AI recommendations.
Required skilled labor support
Skilled labor is essential for both initial setup and ongoing optimization. Data scientists are needed to develop or refine forecasting models, while IT specialists must ensure seamless integration with existing systems. Supply chain managers need to be trained to interpret AI outputs and align them with operational strategies. Over time, the demand for these resources decreases as the system matures.
Current viability/availability
This technology is highly viable and widely available. Leading providers such as SAP, Oracle, and specialized AI firms offer robust forecasting solutions. Advances in cloud computing and machine learning have made these tools more accessible, even for mid-sized manufacturers. However, achieving the full potential of AI forecasting requires an investment in customization and data management.
Use Case 3: Predictive Maintenance
Potential impact on operations
Predictive maintenance routines are great for improving your OT’s overall equipment effectiveness (OEE) and mitigating costly production delays caused by unforeseen malfunctions or unplanned downtime. AI enhances this process by analyzing real-time equipment data and making adjustments as needed—for example, if a system is out of variance, notifications are immediately sent out to key personnel, who then monitor the variance. AI can even shut down the equipment to await a simple repair that prevents a larger problem (and more downtime) from developing. AI-powered tools can fully automate workflows and cleaning cycles, optimizing operations and reducing costs. The improved quality, speed, and consistency AI provides reduces overhead costs and leads to happier customers.
Ease of implementation
Many OEMs offer equipment monitoring tools that can be used for predictive maintenance, such as analyzing grease for ferrous content or using the noise signature of drives to diagnose issues. It is clear that a well-maintained machine will perform longer and likely yield an enhanced ROI through better uptime.
Required skilled labor support
Trained plant maintenance personnel can perform normal routine maintenance on robots and AMRs, which many automated skid systems offer clean-in-place processes. Quite often, plant personnel will collaborate with maintenance experts from the OEM to perform more sophisticated preventative maintenance routines.
Current viability/availability
Predictive maintenance routines are an industry standard for equipment in manufacturing environments. Training for this type of service is readily available, as are opportunities for AI integration into equipment monitoring systems.
Use Case 4: Digital Twins
Potential impact on operations
Digital twin technology is a virtual representation of a product or a physical, operational environment. Depending on the number of sensors deployed to monitor a system, digital twins can be incredibly detailed. Using gathered operational data, AI can analyze hundreds or even thousands of variables that impact system performance, finding the best combination of variables to optimize productivity, supply chain forecasting, and decision making. With AI-driven digital twin technology, new designs and innovations can be tested and vetted in the virtual world before any changes are made to the real-life processes.
Such simulation allows manufacturers to more readily predict a manufacturing outcome or process flow before the automation is released to production. This enables an accurate assessment of success or bottlenecks, taking the guesswork out of a predicted outcome and preventing delays and costs associated with system redesign.
Ease of implementation
Several digital-twin packages incorporate accurate kinematic models of various robots and AMRs. This allows for more accurate representation of what a programmed task will look like, including input/output communications that might emulate the operation of machinery. Some available packages have libraries of various robot and AMR brands that help users build customized simulation models.
Required skilled labor support
When deploying digital-twin simulations, it is best to employ the talents of people who understand CAD, 3D modeling, mechatronic relationships, and programmable logic controller (PLC) basics. Such a team is better prepared to understand how the software model is programmed and its ultimate relationship to the actual machine environment.
Current viability/availability
Several producers of software platforms and OEM equipment provide digital-twin capabilities, allowing for operational cause-and-effect as well as operational readiness checks and simulation.
Looking Ahead
As manufacturers gain knowledge about AI and what it can do, they realize the importance of integrating AI into their manufacturing processes to stay competitive in achieving speed to market and meeting customer demands for quality. The combination of AI with digital twins and generative design can quickly identify innovative designs and manufacturing approaches that will increase customer satisfaction, boost market share, and reduce operational costs.
Some opinions expressed in this article may be those of a contributing author and not necessarily Gray.